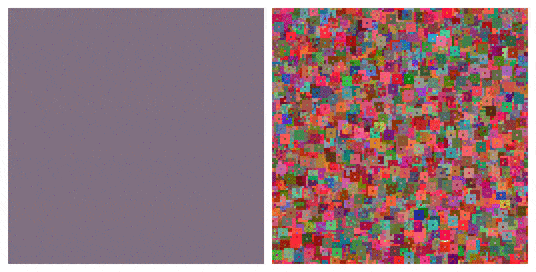
Dynamics after evolution of the genetics: explained more at 9:20 in the video below.
Summary
- GoodAI deepens its explorations into evolving AI architectures with a new paper — Bootstrapping of memetic from genetic evolution via inter-agent selection pressures — on patterns of meme transmission within populations of agents.
- The study traces memetic evolution to understand its interplay with genetic evolution and specifically, whether open-ended learning algorithms can be discovered through the lens of socio-cultural learning models.
The backdrop of GoodAI’s latest research intersects cultural evolution and memetics discourse. Drawing on Dual Inheritance Theory [1.2] and Richard Dawkins’ concept of meme [3], Nicholas Guttenberg and Marek Rosa investigate how message exchange in a population of agents with genetic predispositions can reveal new learning algorithms through a socio-cultural learning paradigm.
Central to their work is the definition of meme as a cultural replicator, a unit of imitation or communication. The idea of meme as a vehicle for cultural inheritance understands human cultural evolution as being comparable to the biological evolution of species. Like genes, cultural memes obey the principles of struggle for survival, natural selection, and heredity.
However, in memetics, memes are not subservient to genes but evolve alongside them, using the biological vehicles constructed by genes as their copying machinery. Like genes, memes are selfish replicators that spread for their own benefit and not necessarily for the benefit of the hosts who copy them, or the culture they form a part of.
Theoretical analyses show that gene–culture co-evolution can be complex and unpredictable. Depending on the environment, cultural traits, like genes, can be more or less adaptive and spread accordingly. If a certain behavior or feature can be either innate or culturally learned, the question arises, what are the advantages of social transmission?
Open-ended Learning
A long-standing goal within artificial intelligence research is the development of open-ended learning systems. Guttenberg and Rosa investigate the possibilities through memetics with the aim to build AI agents capable of setting meaningful goals for themselves. While standard genetic and deep learning AI systems tend towards homogeny, structural and behavioral diversity are key components for an open-ended learning AI. Experiments suggest that collective learning and memetics can be leveraged to develop such systems.
Nicholas Guttenberg talks about the paper at the 2021 ALIFE conference.
Closely coupled with open-ended learning and self-invented goals is the concept of self-improvement. Questions underlying the paper address the ability of artificial organisms to evolve into better hosts for their memes and how memes could evolve to improve the circumstances of their host organisms. If memetic variations influence the genetic evolution of the agents, memes could drive their hosts towards becoming better at propagating memes.
Memetic evolution might as well serve to improve the abilities of genetic evolution by smoothing the landscape of genetic adaptation and making learning easier. Called the Baldwin effect [4], this occurs when organisms adapt in non-heritable ways within their own lifetime. If a genetic change leads to a limb becoming longer, an adaptive nervous system can adjust the limb’s control policy on the fly. The organism’s chances of survival increase: if a behavior is learned during its lifetime, it doesn’t have to rely on a simultaneous genetic change of its form and behavior to maintain or improve fitness.
Adaptation during lifetime (or in other words, at agent’s test-time, without retraining) can be viewed as an important feature of open-ended learning systems, resulting in flexible learners who gradually accumulate their skills without forgetting previous knowledge.
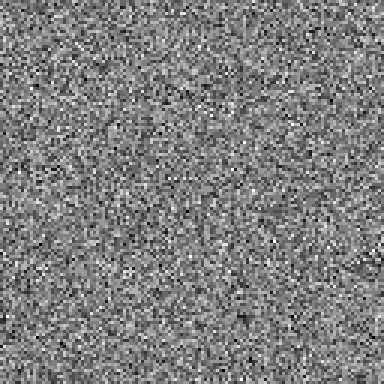
Cooperation vs defection in a memetic Iterated Prisoner’s Dilemma with some subtle phases emerging.
Findings and Observations
Guttenberg and Rosa set out to characterize the interplay between meme transmission and genetic pressures. To address the benefits or drawbacks of a fast cultural mode of adaptation in the presence of a slower genetic mode, they create a population of agents capable of message transmission and observe which messages survive and get replicated. In such a setting, there isn’t one fitness function. On the contrary, there are as many fitness functions as there are agents with each expressing their own preferences for certain messages.
Study findings highlight the following noteworthy characteristics:
- Rather than a single ’most fit’ meme dominating for all time, the study witnessed an emergent diversity in the space of memes during the continual production of new memes alongside the survival of particular older ones. While not a sufficient condition to produce an open-ended learning system, it is likely a necessary one.
- Continual replications of memes and the ongoing production of new ones relied on agent-provided selection pressure. While a population of identical agents was sufficient to obtain a small number of high-population memes, results demonstrating diversity were only observable when agent preferences for memes translated into replicative pressure.
Final Thoughts
Socio-cultural learning theories and memetics hold keen promise for future investigations into continual modes of knowledge transfer and learning. Guttenberg and Rosa’ interdisciplinary approach open novel lines of inquiry into the development of self-guided, lifelong-learning AI capable of fast adaptation to new problems on the fly, without retraining (what we call infinite inner loop learning).
The GoodAI team has been looking for inspirations on how infinite inner loops occur in natural systems (as opposed to the evolutionary outer loop learning). Memetics as a mechanism of cultural transmission provide a compelling example. Memes aren’t closely coupled with individual organisms’ fitness, allowing them to jump over evolutionarily disadvantageous valleys and gradually accumulate and evolve in the adaptive cultural layer. A powerful learning mechanism in collectives — the replication of messages, or memes — allows members of a collective to influence each other at a distance in both space and time. One might argue that memetic transmission can exhibit features of goal-directed intelligence.
GoodAI’s joint research framework — the Badger architecture — is a collective of interacting units. Its modularity departs from traditional deep learning systems’ monolithic nature and serves as a convenient substrate for memetic explorations. This study joins GoodAI’s growing repertoire of work with Memetic Badger. If you would like to collaborate on interdisciplinary research into collective computation, systems evolving through culture, and lifelong learning AI, get in touch with us to join our team or check out GoodAI Grants to apply for funding.
For the latest from our blog sign up for our newsletter.
Source code for the simulations: https://github.com/GoodAI/memes
Original paper: https://arxiv.org/pdf/2104.03404.pdf
References
[1] Cavalli-Sforza, L. L. and Feldman, M. W. (1981). Cultural transmission and evolution: A quantitative approach. Princeton University Press.
[2] Boyd, R. and Richardson, P.J. (1988). Culture and the evolutionary process. University of Chicago Press.
[3] Dawkins, R. (1976). The Selfish Gene. Oxford University Press
[4] Baldwin, J. M. (1896). A new factor in evolution (continued). The American Naturalist, 30(355):536–553.
Nice Article! Can’t wait for your next blog.
Thank you!